AI for Parkinson Lab
A collaboration between Verily, Radboud UMC, Radboud University, and the Donders Institute at Radboud University.
The AI for Parkinson Lab is researching the use of AI-based tools to process raw wearable sensor and video data into digital biomarkers to monitor Parkinson’s disease in real-life, in order to provide more reliable and sensitive assessments than current clinical assessments. The AI for Parkinson Lab is a collaboration between Radboud UMC, Verily, Radboud University, and the Donders Institute at Radboud University.
Parkinson’s disease (PD) is a rapidly growing neurological disorder worldwide, with an estimated doubling of patients to 12 million by 2040. As a progressive condition, PD leads to severe impairments in the late stages, and novel treatments to slow or halt the underlying neurodegeneration are being developed. However, testing their efficacy is hindered by the lack of sensitive and reliable endpoints. Currently, the progression of PD is mainly evaluated by clinical experts who measure the symptom severity when patients visit the clinic, but this method is limited by intra- and inter-rater variability, as well as observer effects and common response fluctuations associated with symptomatic medication.
Our vision is that remote monitoring, combined with trustworthy AI, has the potential to transform clinical trials and care for people with Parkinson’s disease, by providing objective and frequent insights into how patients function in their home environment. The AI for Parkinson Lab will focus on two different systems for remote monitoring: wearable sensors and video recordings. Both systems can be used to capture hallmark motor (e.g., tremor, bradykinesia, and gait impairments) and non-motor symptoms (e.g., sleep, autonomic impairments) of PD. The challenge is to extract reliable information about these PD symptoms from the heterogeneous real-life raw sensor signals, which not only reflect PD-specific signs but also behavioral and environmental factors.
We now have access to multiple worldwide unique datasets which include wearable sensor data and video recordings of large groups of PD patients. An important example is the Personalized Parkinson Project (PPP), which originated from the existing collaboration between Verily Life Sciences and Radboudumc. PPP is a single-center cohort study including 550 early PD patients who are followed for up to 3 years. In addition to elaborate annual in-clinic assessments (including expert evaluation of symptoms, video recordings, fMRI, ECG, and collection of biosamples), patients are monitored in real-life using a multi-sensor smartwatch, which continuously collects raw sensor data from multiple sensor types during the full 3-year follow-up period.
The AI for Parkinson Lab aims to use this and other datasets to develop AI-based tools to process raw wearable sensor and video data into digital biomarkers to monitor Parkinson’s disease in real-life. We will investigate whether such biomarkers are indeed more reliable and sensitive to change compared to current clinical assessments and to optimally use a large amount of unlabeled data, we will investigate the value of promising developments in unsupervised and semi-supervised deep learning. To support the applicability of the developed tools, we will investigate the perspective of patients, clinicians, and other stakeholders (e.g. regulatory bodies, pharmaceutical industry) during all stages of the projects.
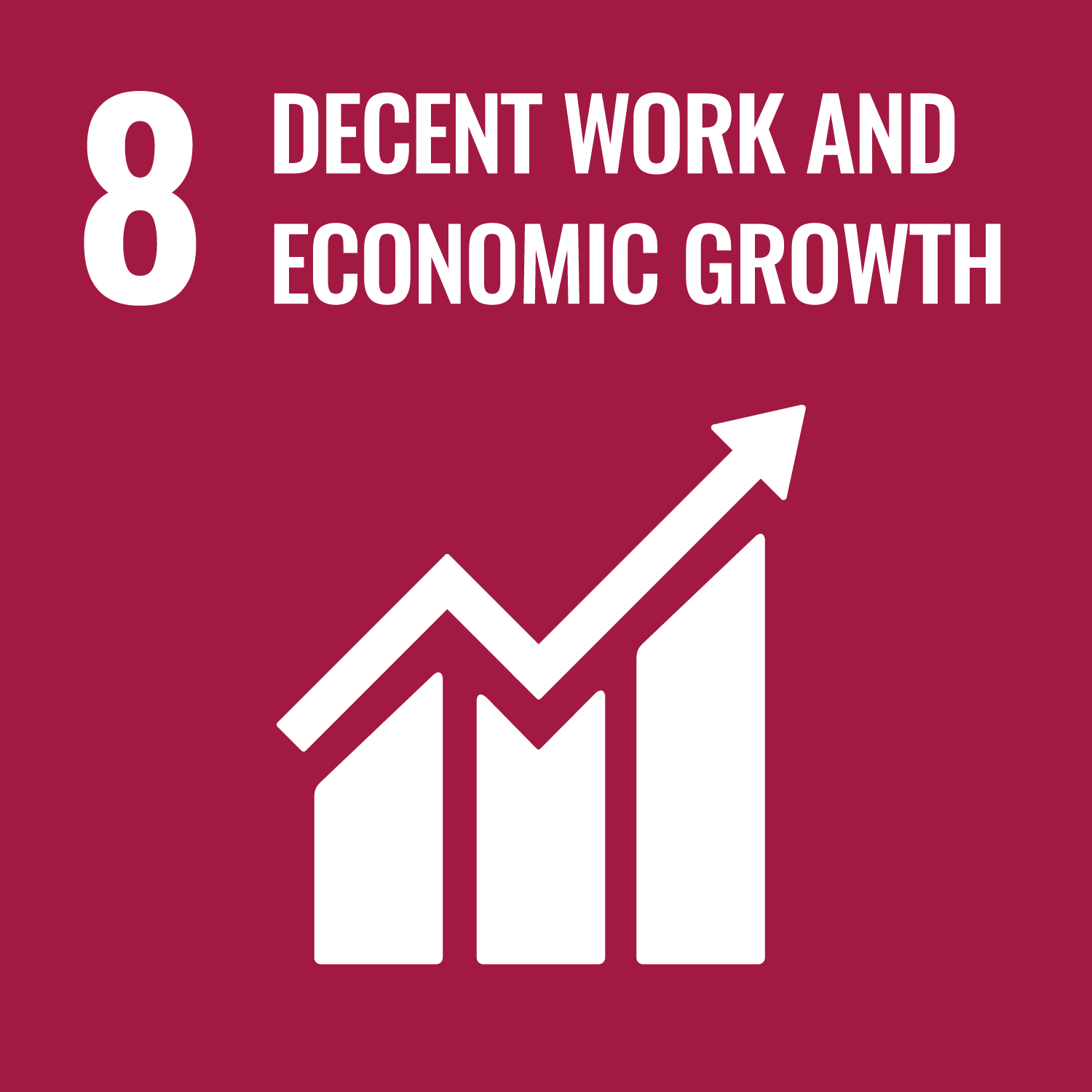
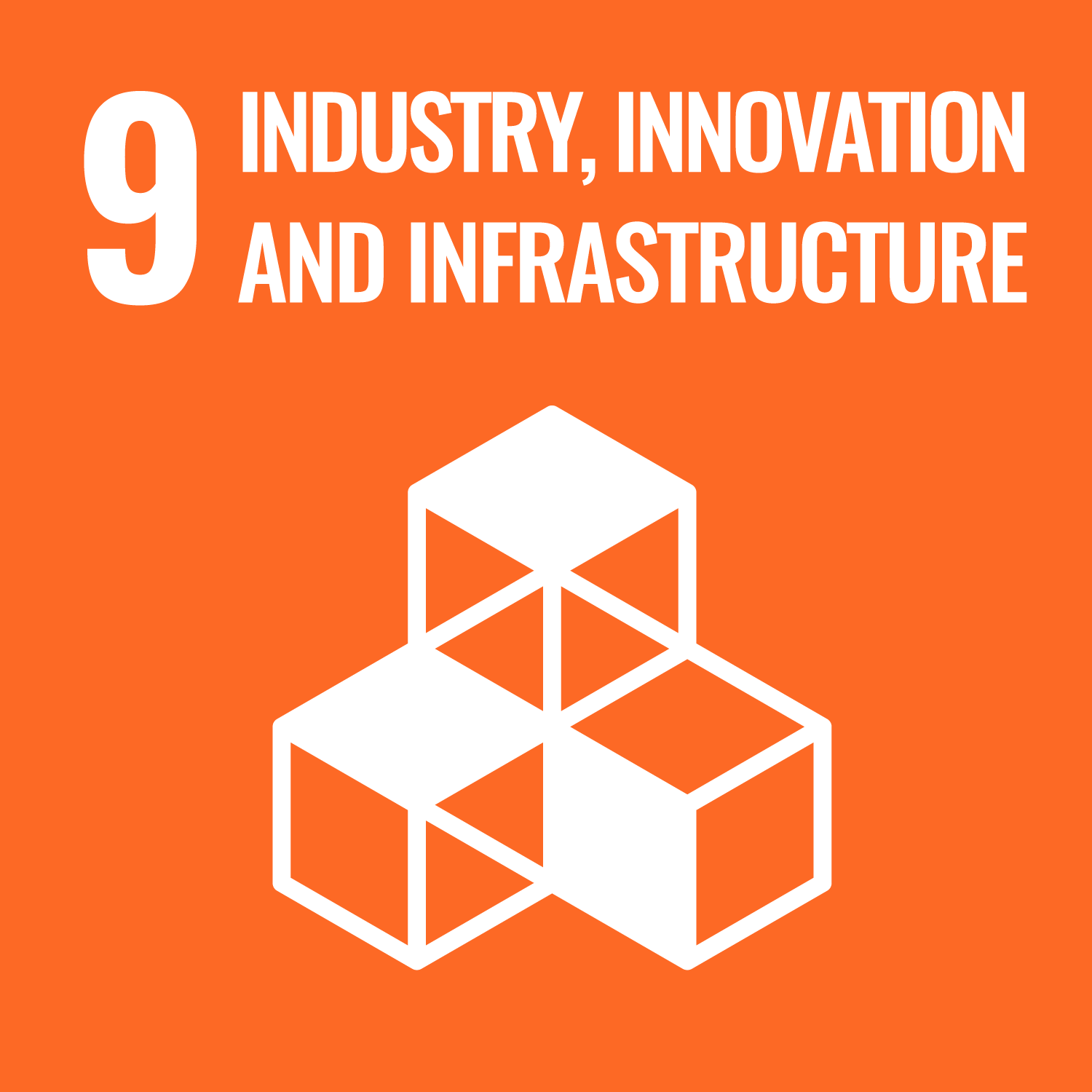
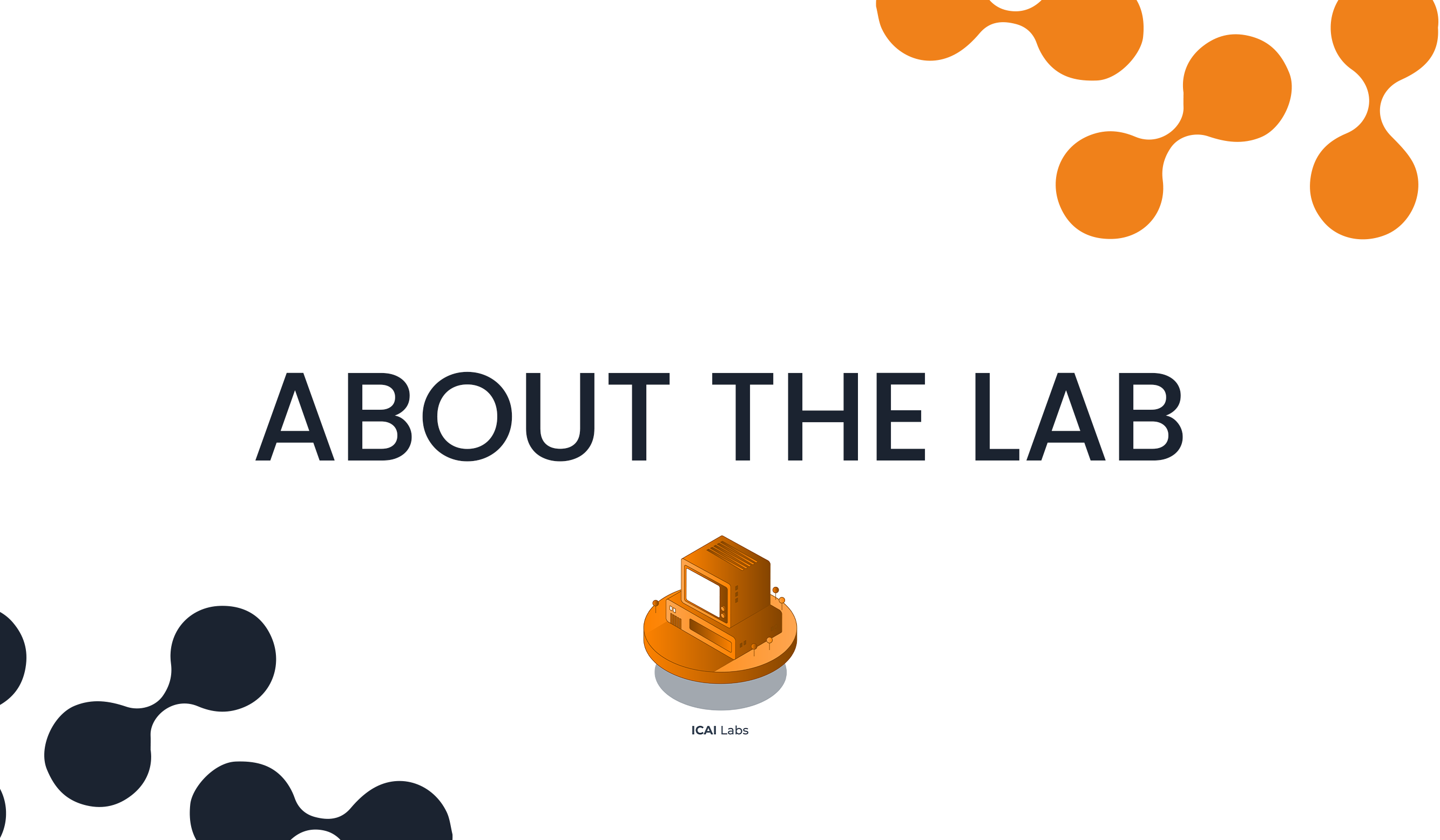
Research projects
Digital progression biomarkers based on real-life monitoring. Aims to determine if and how real-life monitoring using a wrist-worn sensor can be used for Parkinson’s disease (PD) motor progression.
Digital progression biomarkers based on real-life monitoring. Aims to determine if and how real-life monitoring using a wrist-worn sensor can be used for PD non-motor progression.
Relationship between clinical and pathophysiological subtypes and PD progression. Aims to use digital progression biomarkers to predict disease progression.
AI-based video analysis for differential diagnosis. Aims to use video AI for differential diagnosis of movement disorders and estimating the severity of PD motor symptoms.
Trust in AI-based remote monitoring tools for PD research & self-management. Aims to understand which conditions need to be satisfied to justify user trust and acceptability.
People
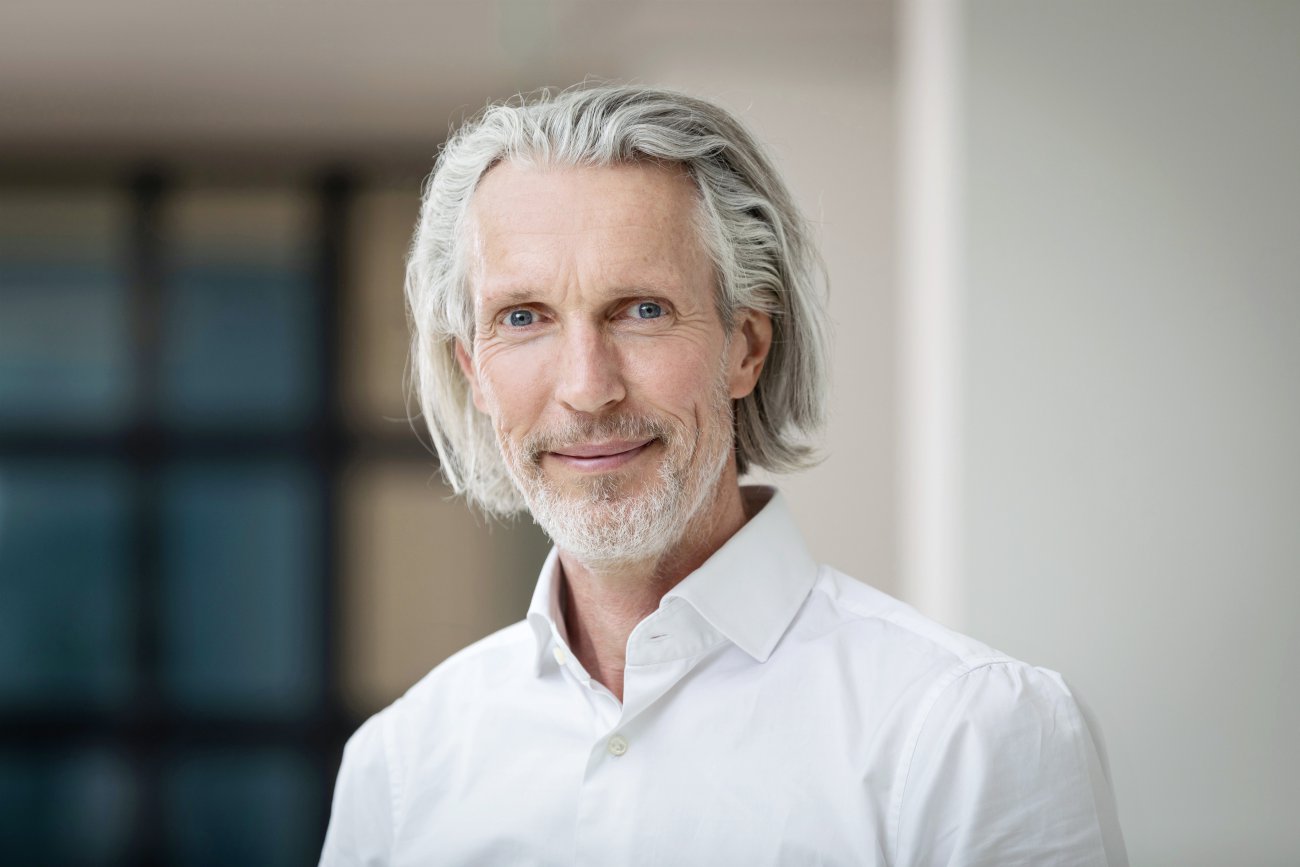

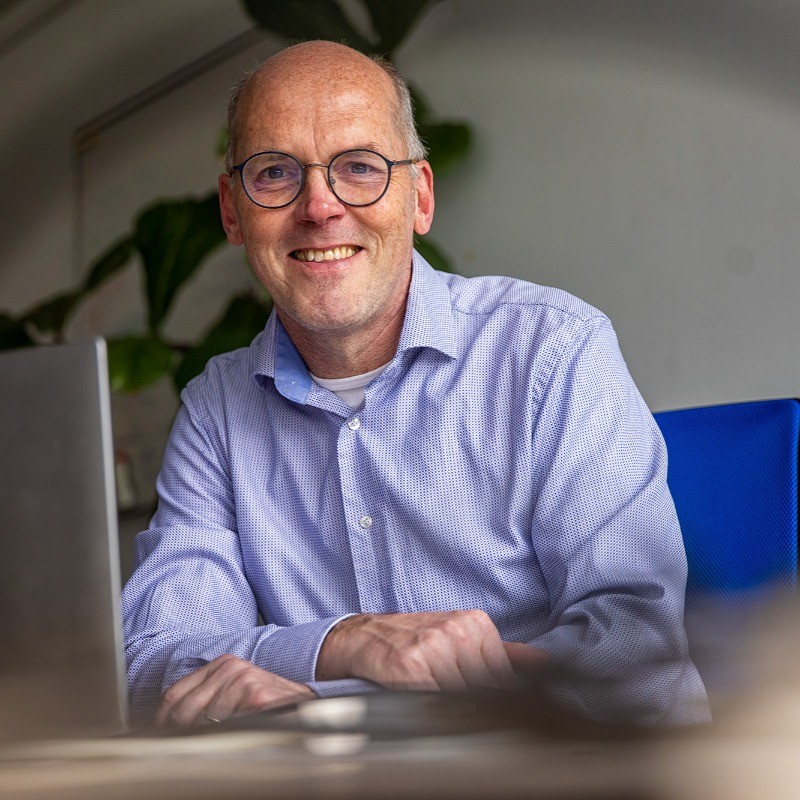
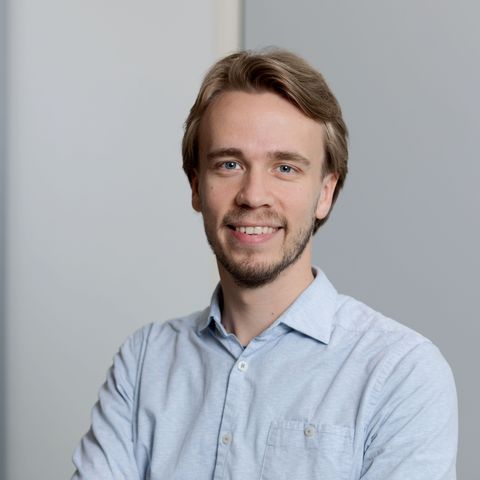
PHD Students
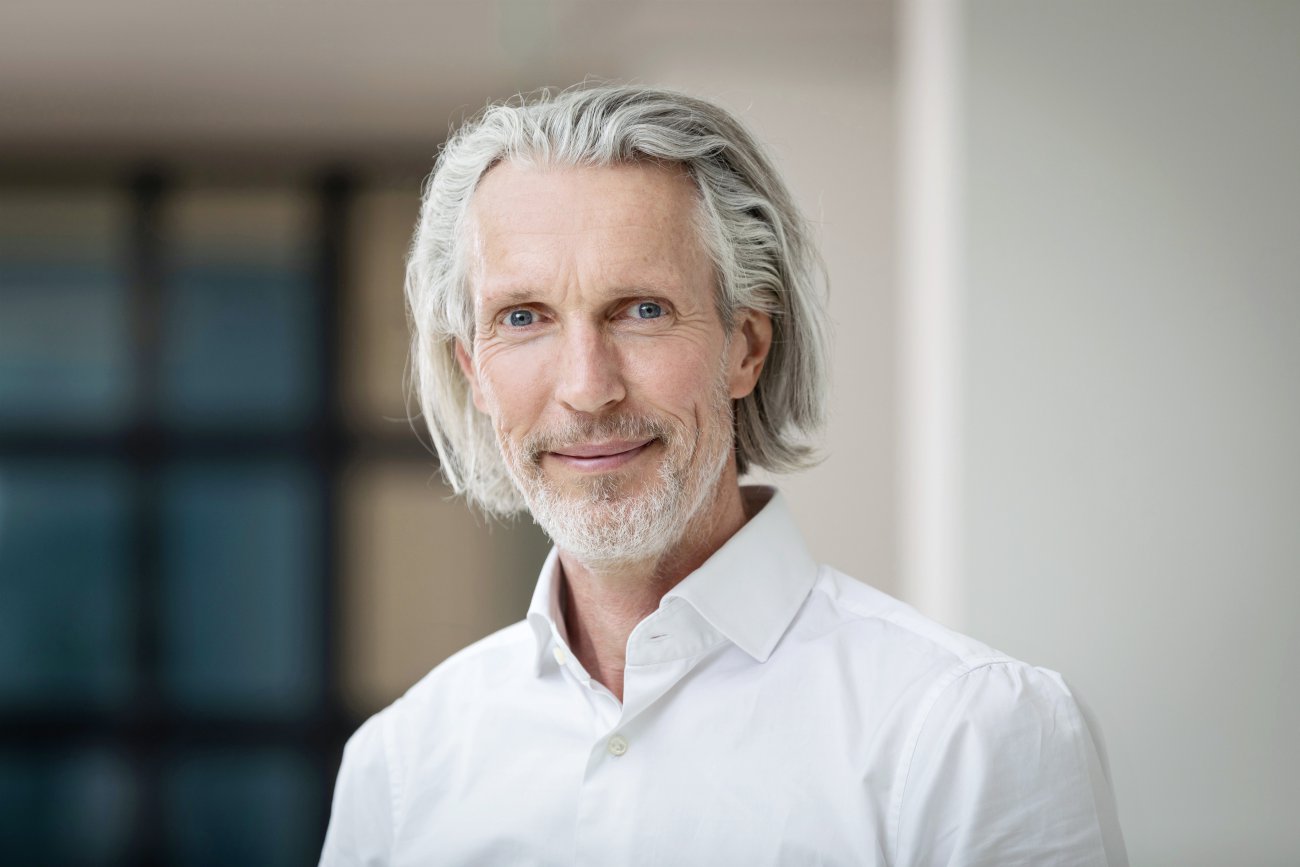

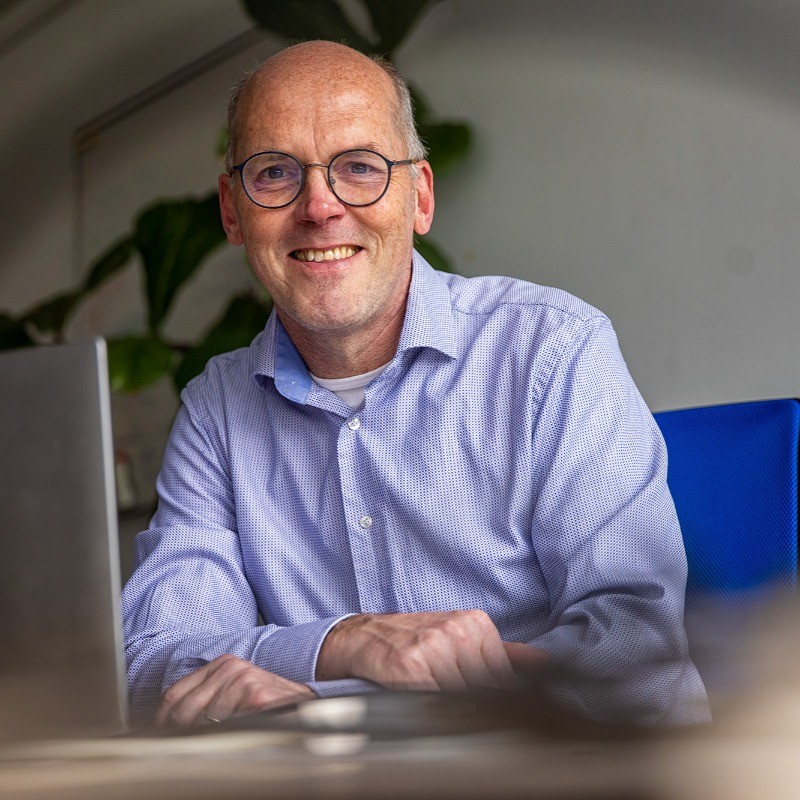
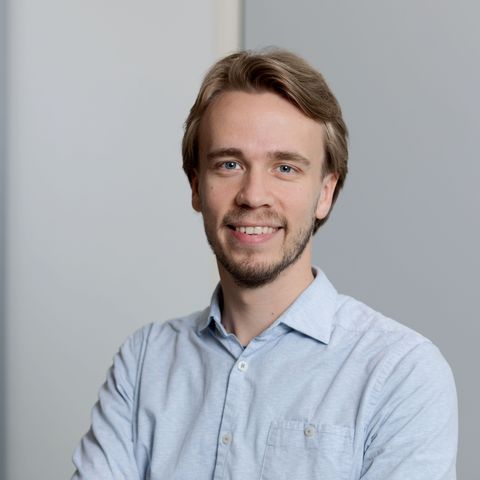
Partners
Radboud UMC
Verily
Radboud University
Donders Institute at Radboud University
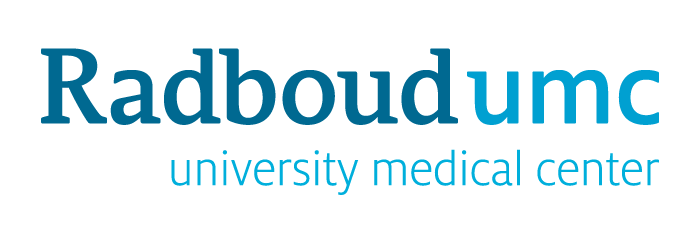
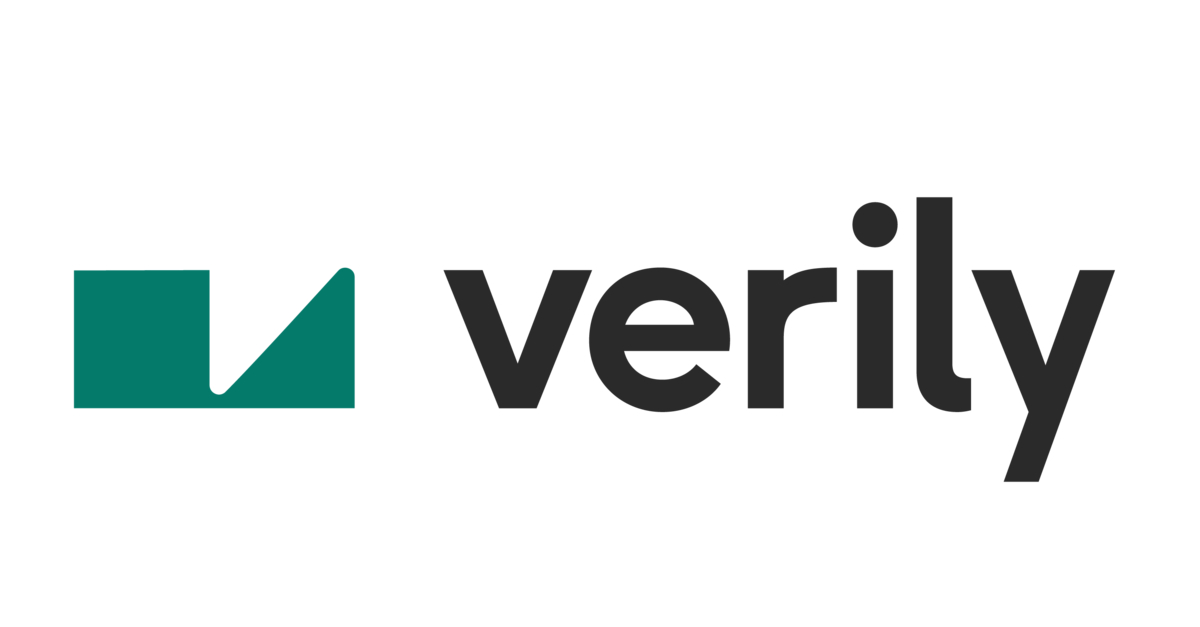
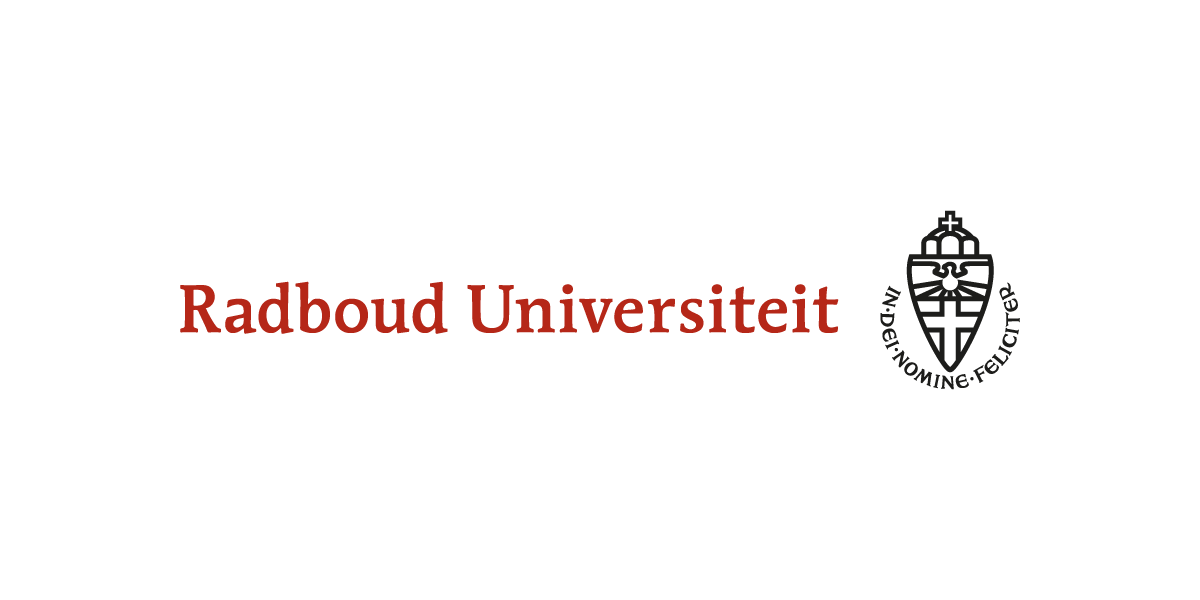
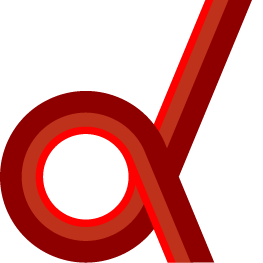