QUVA Lab
A collaboration between Qualcomm and the University of Amsterdam.
The mission of the QUVA-lab is to perform world-class research on deep vision. Such vision strives to automatically interpret with the aid of deep learning what happens where, when and why in images and video. Deep learning is a form of machine learning with neural networks, loosely inspired by how neurons process information in the brain. Research projects in the lab focus on learning to recognize objects in images from a single example, personalized event detection and summarization in video, and privacy preserving deep learning. The research is published in the best academic venues and secured in patents.



Research projects
Adaptable Foundation Models (Danilo de Goede): Foundation models have established themselves as a revolutionary class of general-purpose AI models that provide impressive abilities to generate text, images, videos, and more. In this project, we study, develop, and evaluate new adaptive learning schemes throughout the foundation model lifecycle, covering pre-training, adaptation, and deployment.
Unsupervised Learning for Source Compression (Natasha Butt): Learned compression has seen recent advantages relative to traditional compression codecs. In this project, we will explore new methods for lossless and lossy compression with unsupervised learning.
Federated Learning (Rob Romijnders): Future of machine learning will see data distributed across multiple devices. This project studies effective model learning when data is distributed and communication bandwith between devices is limited.
Efficient Video Representation Learning (Mohammadreza Salehi): Despite the enormous advances of image representation learning, video representation learning has not been well explored yet because of the higher computational complexity and the space-time dynamics shaping the content of a video. In this research, we try to capture the content of a video more efficiently in different aspects such as data and computational efficiency.
Video Action Recognition (Pengwan Yang): In this project, we focus on video understanding with the goal of alleviating the dependency on labels. We develop methods that leveraging few-shot, weakly-supervised, and unsupervised learning signals.
Hardware-Aware Learning (Winfried van den Dool): We study and develop novel approaches for hardware-aware learning, focusing on actual hardware constraints, and work towards a unified framework for scaling and improving noisy and low-precision computing.
Generalizeable Video Representation Learning (Michael Dorkenwald): In this project we aim to develop self-supervised methods that obtain generalizeable video representations and solve novel tasks for which the usage of multiple modalities and is a necessity, such as video scene understanding.
Geometric Deep Learning (Gabriele Cesa): Many machine learning tasks come with some intrinsic geometric structure. In this project, we will study how to encode the geometry of a problem into neural-network architectures to achieve improved data efficiency and generalization. A particular focus will be given to 3D data and the task of 3D reconstruction, where the global 3D structure is only accessible through a number of 2D observations.
PHD Students



Partners
Qualcomm is a research and product development organisation in Amsterdam. It is the world’s leading wireless technology innovator and the driving force behind the development, launch, and expansion of 5G.
University of Amsterdam (UvA) is the Netherlands’ largest university, offering the widest range of academic programs.

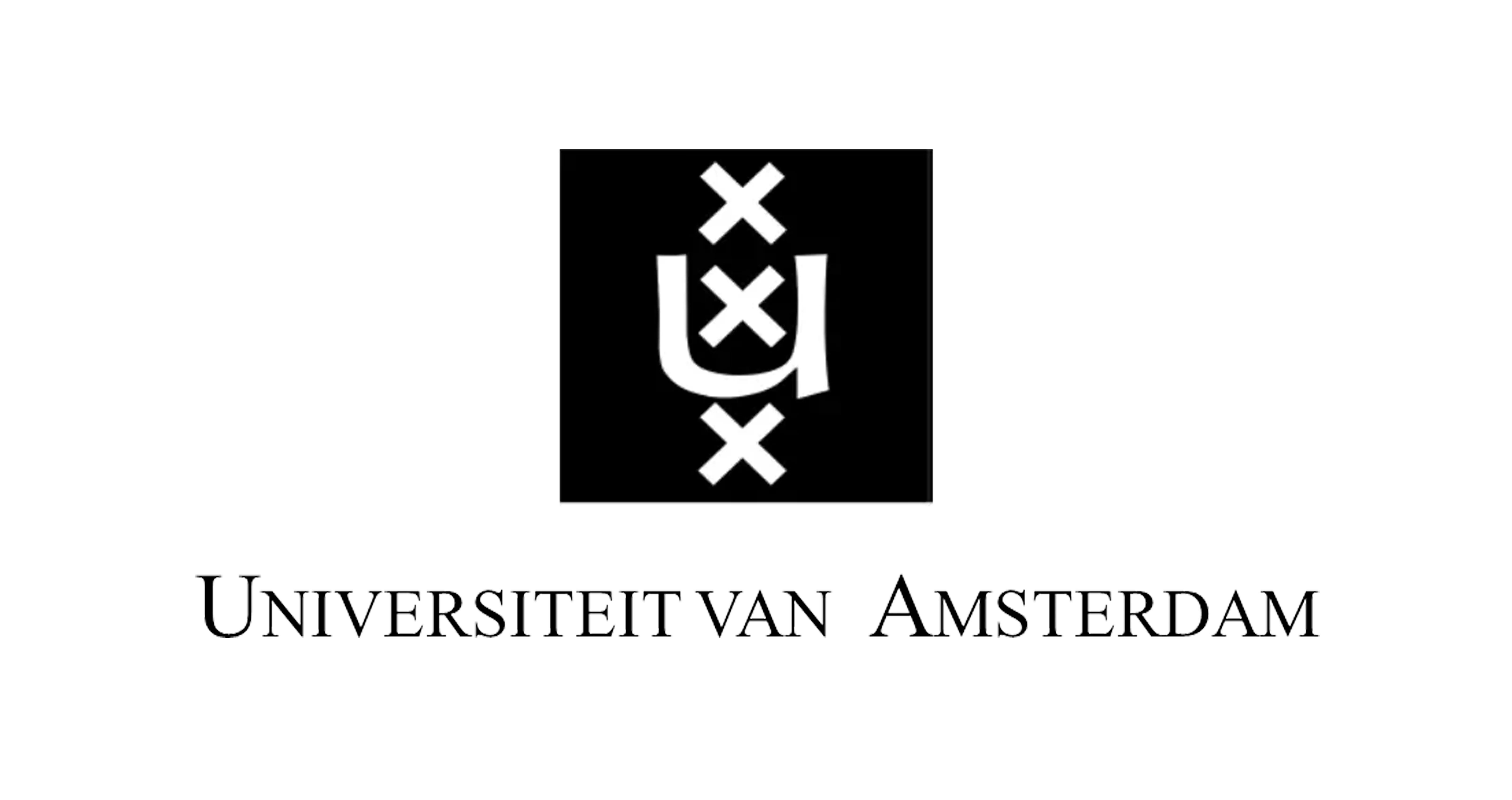