AI in Healthcare – Moving from Bytes to Bedside
The adoption of artificial intelligence (AI) in healthcare is progressing at an unprecedented pace, driven by the increasing demand for better patient outcomes, workforce challenges, and advancements in data and technology. However, despite the buzz, significant hurdles remain in operationalizing AI at the clinical level.
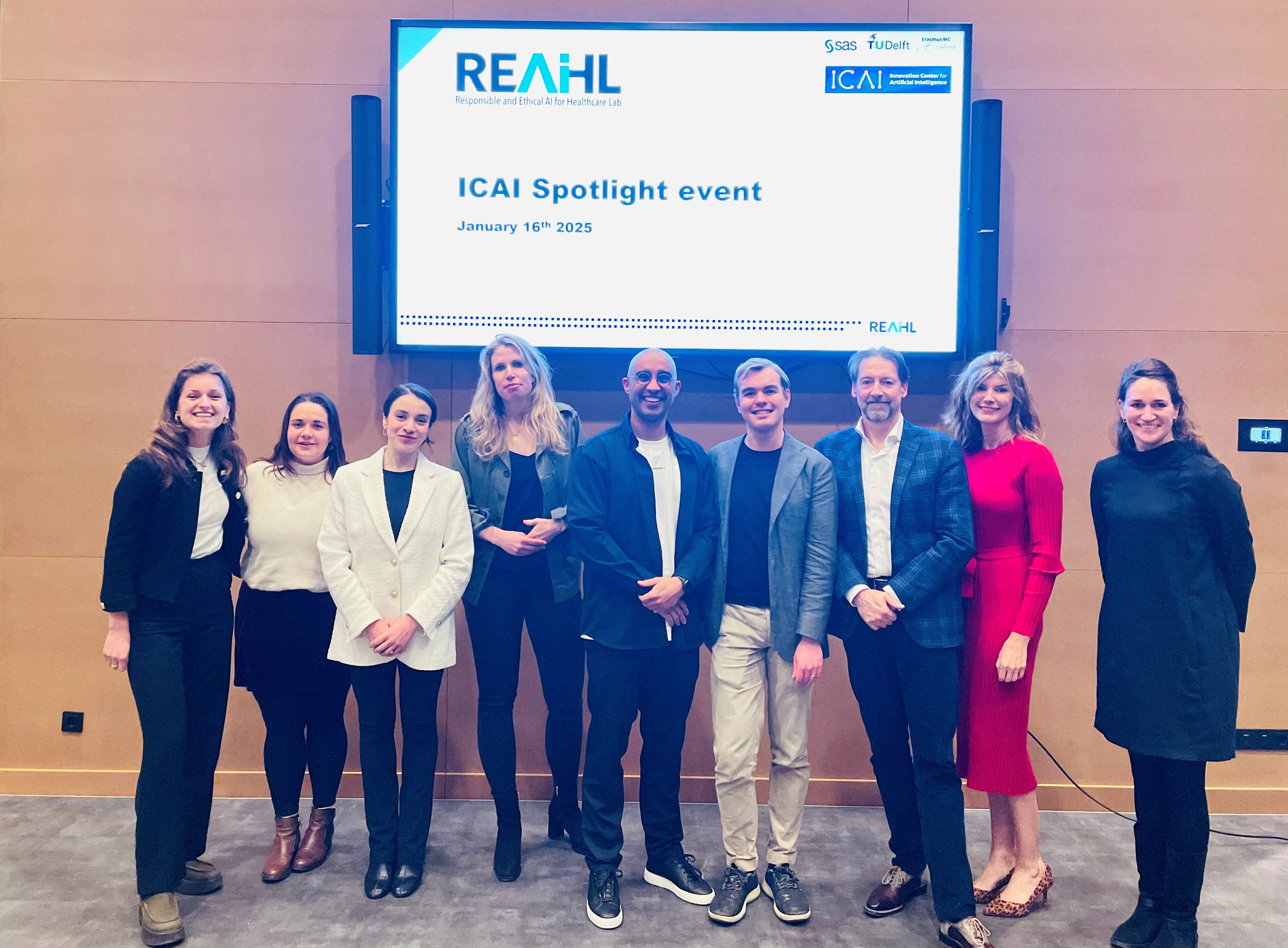
Insights from the REAiHL Spotlight Event
At the recent ICAI Spotlight event featuring the REAiHL Lab project, dr. Michel vanGenderen of Erasmus MC delivered an insightful presentation on this topic, shedding light on the challenges and opportunities of implementing AI in healthcare.
The Responsible and Ethical AI for Healthcare Lab (REAiHL) is a collaboration between SAS, Erasmus Medical Center, and Delft University of Technology. The ICAI endorsed lab's mission is to conduct research, design and implement AI systems, and translate the World Health Organization (WHO) ethical principles into clinically (and technically) feasible principles that can guide the development and deployment of AI technologies in healthcare. Through synergistic efforts, academic stakeholders (Erasmus MC and TU Delft) and non-academic stakeholders(SAS, global leader in data and AI) are fostering collaboration and innovation to advance the ethical use of AI in healthcare.
Challenges in Healthcare AI
Healthcare professionals face a growing administrative burden, leading to burnout and staffing shortages. Although AI offers promise, the reality is sobering: most AI models remain in the prototyping phase, with less than 2% integrated into clinical workflows. Achieving bedside implementation remains elusive, underscoring the need for a strategic shift.
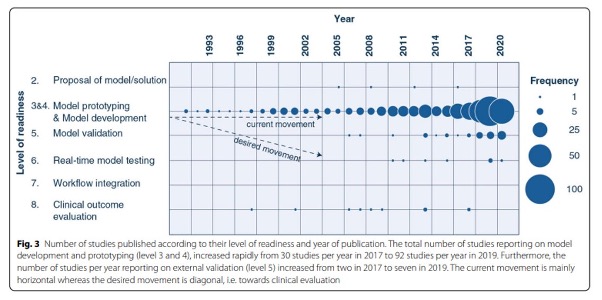
AI adoption, particularly in ICUs, remains limited due to bias, insufficient datasets, and lack of transparency. According to this paper "AI hesitancy" has significant costs, including poorer patient outcomes and inefficient resource use. Addressing these challenges is crucial to move “from bytes to bedside,” unlocking AI’s potential to transform patient care.
The Potential of AI and LLMs
Large Language Models (LLMs) like GPT-4 have demonstrated remarkable capabilities, achieving a 90% pass rate on medical licensing exams. These models do not replace clinicians but offer tools to enhance decision-making, providing better insights when used responsibly. Yet, the rapid rise in AI-related ethical controversies highlights the risks of misuse, as evidenced by a 26-fold increase in reported incidents since 2012 (Artificial Intelligence Index Report2023).
Bias in AI: A Persistent Challenge
Bias in AI systems poses a critical challenge. A study by Obermeyer et al. revealed that algorithms predicting healthcare needs based on past costs, rather than health indicators, exacerbated disparities in care. Black patients, historically underserved due to systemic inequities, were disproportionately affected. By correcting for bias, the percentage of Black patients receiving early care increased significantly, from 17.7% to 46.5%.
Bias arises from three sources: historical data, the data itself, and human interpretation. As the adage goes, "bias in = bias out." To mitigate this, diverse and representative datasets, ethical data collection, and standardized metrics to evaluate algorithmic fairness are essential. The creation of AI registries and model cards—tools that log model design, data, and performance metrics—can further enhance transparency and trust.
WHO Principles for Ethical AI
Stefan Buijsman highlighted the importance of adopting the World Health Organization’s(WHO) principles for AI in healthcare, which prioritize transparency, fairness, inclusivity, and accountability. While it is technically impossible to create completely unbiased models, understanding and addressing biases, such as those related to ethnicity, is critical to ensure fair and effective decision-making.
Despite their significance, less than half of original healthcare AI studies incorporate these ethical principles. Designing AI systems with consequential values—such as autonomy, competence, and authenticity—bridges the gap between ethical ideals and practical application, aligning AI with the needs of both patients and clinicians.
Putting Values into Practice
In its first year, REAiHL has focused on mapping the needs and values for AI in healthcare. The goal is to identify what ethical principles matter most to doctors and other stakeholders and translate these values into actionable design requirements. The key value:
- Autonomy: Empowering users and patients. It can be translated into design requirements by looking at the different components of autonomy, often seen as self-governance—the ability to achieve personal goals. Both must be protected.
- Competence: Doctors and patients can achieve their goals, based on their own values.
- Authenticity: Doctors and patients values are authentic, so not unduly influenced by the AI systems they interact with
How do we ensure AI design truly reflects the values we aim to uphold? The key lies in making values design-consequential—shaping not only the systems we build but also the choices they enable.These dimensions guide actionable design decisions. For example, users can be provided with the right information to judge when to follow AI recommendations or opt for alternative actions.Similarly, large language models (LLMs) can help elicit patients’ values, ensuring these are better integrated into decision-making. By embedding values like autonomy into AI systems, we move closer to creating tools that genuinely empower and serve human needs.
Moving Towards Responsible AI
AI in healthcare requires a systemic approach, much like a Formula 1 racing team where every component must function seamlessly. Data strategies, robust infrastructure, and responsible AI practices form the foundation for success. Initiatives like REAiHL exemplify the collaborative efforts needed to move AI from the lab to the bedside.
The Path Forward
Operationalizing AI in healthcare demands continuous evaluation and adaptation. From addressing data bias to fostering cross-disciplinary collaboration, the journey from bytes to bedside is a complex but necessary endeavor. With a focus on responsible implementation, we can ensure AI enhances the human touch, ultimately improving patient care and outcomes.The REAiHL initiative shows that integrating ethical considerations, fostering trust, and emphasizing inclusivity are key to ensuring a responsible approach to healthcare innovation. These efforts move beyond theoretical discussions, translating principles into actionable strategies that truly benefit patients and clinicians alike.