The importance of privacy-focused engineering in AI modeling pipelines at KPN
The month November was dedicated to the KPN Responsible AI Lab. Three of the PhD students in the lab, Henry Maathuis, Niels Scholten and Nemania Borovits gave insights into their backgrounds and the research they employ within the lab:
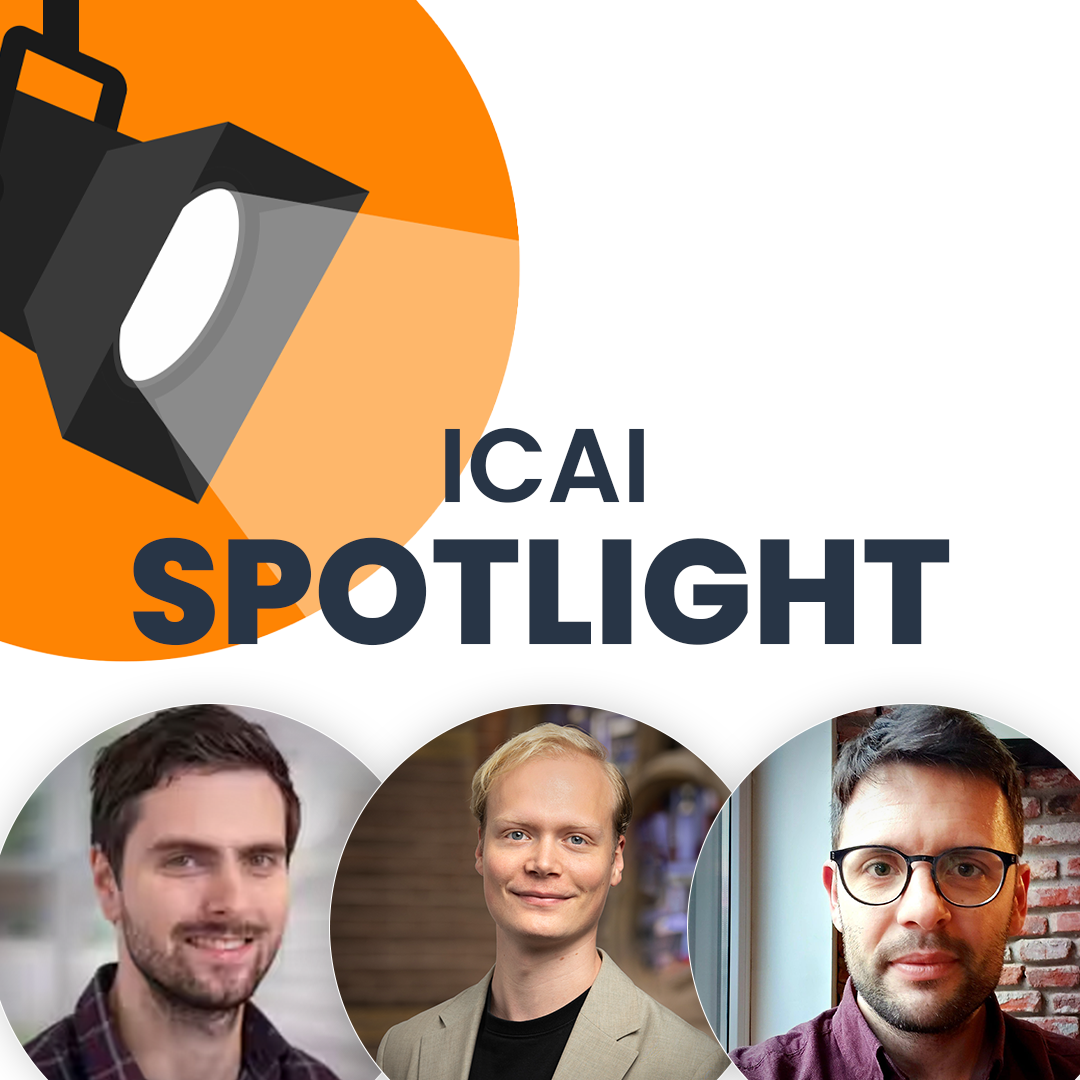
Nemania Borovits
“A few years ago, I worked as a software and data engineer in Greece, focusing on industry-driven projects. After five years in this role, I decided it was time to challenge myself further and explore my interest in data science more deeply. This decision led me to the Netherlands, where I enrolled in the DataScience & Entrepreneurship program. During these studies, I discovered anew passion: scientific research. This realization opened my eyes to the potential of data science, not only as a powerful tool for industry but also asa force for societal good.
Completing my master’s program, I found myself at a pivotal moment—ready to apply my skills in data science while making a meaningful impact. I found this unique opportunity within the KPN Responsible AI Lab of ICAI, where I could combine my industry experience with academic research, focusing on Data Engineering and AI for Privacy. Privacy engineering, especially in the realm of AI, was are latively new field, gaining momentum with the formal introduction of the GDPR in 2018. The chance to contribute to this emerging field, particularly in areal-world industry context, was incredibly appealing to me.
When I began my PhD journey, I quickly realized the importance of privacy-focused engineering in AI modeling pipelines at KPN. This process, however, was not without its challenges. Privacy engineering as a scientific discipline is still evolving, and implementing it effectively requires close collaboration across diverse teams—from engineers to legal counsels and Data Privacy Officers.Through these collaborations, we not only integrated privacy into various AI solutions within the KPN ecosystem but also managed to share our work with the broader research community by publishing in rank A scientific conferences and Q1 journals.
Privacy has been identified by Forbes as one of the most critical challenges of our decade.While non-compliance poses legal risks, many industry leaders, including IBM,Amazon, Microsoft, and others, have recognized that the real competitive advantage lies in building societal trust by transparently engineering privacy into their IT and AI solutions. At KPN, this vision of fostering trust through privacy-centric design is a driving force behind our work. Collaborating with industry experts who share this commitment has enriched my PhD journey, allowing me to contribute to scientific advancements in privacy-preserving AI while addressing real-world challenges.
Through this experience, I’ve come to appreciate the unique value of combining academic research with industry practice. It’s not only about meeting regulatory requirements; it’s about building AI solutions that people can trust, knowing that their privacy is prioritized at every step. I’m excited to see how our work continues to influence both the academic field and the broader industry, setting new standards for privacy-preserving AI.”
-------------------------
Henry Maathuis
Henry Maathuis is pursuing a PhD at the ICAI Lab for Responsible AI, a collaboration between KPN, Hogeschool Utrecht and JADS. He focuses on improving the detection of cable breaks in telecommunications networks. His research addresses a key challenge faced by operators: distinguishing between cable breaks and transient errors, like power outages, which can trigger similar alarms. Accurate identification of cable breaks is essential for reducing downtime and ensuring reliable service for customers.
Currently, KPN relies on predefined decision rules to analyze alarms generated by network devices. These devices monitor cables and trigger alarms when potential issues arise. There are unfortunately limitations to using decision rules: their predictive performance is limited and are specifically tailored to the current networking devices. The former means that transient errors are sometimes misidentified as cable breaks, leading to unnecessary interventions or, conversely, overlooked cable breaks that result in longer disruptions. The latter means that the decision rules might no longer work when certain network devices are replaced in the future.
Henry’s research focuses on analyzing how alarms behave across the network. Cables are connected through multiple network devices, and when an issue occurs, it triggers a pattern of alarms throughout the system. Different types of issues —such as cable breaks or power outages — lead to distinct alarm patterns. By analyzing these patterns collectively, rather than in isolation, the goal is to improve the accuracy of identifying cable breaks.
To address the limitations of decision rules, Henry is exploring more powerful models, particularly graph neural networks (GNNs). These models are ideal for analyzing complex systems like telecommunications networks. By learning from how alarms propagate through the network in a graph structure, the GNN can detect patterns that signal true cable breaks versus less critical events, such as power outages.
A key aspect of Henry’s research is making the GNN’s predictions explainable. By using explainability techniques, operators can understand how the model arrives at its conclusions. This transparency allows network operators to see which alarms and patterns are most strongly associated with cable breaks. With this knowledge, they can make more informed decisions about when to send out mechanics and when an issue is likely due to something transient, like a power outage.
The goal is to modernize network management at KPN, making it more efficient and reliable. By using advanced models like GNNs and focusing on explainability, his work has the potential to significantly reduce network downtime, improve decision-making for operators, and ultimately provide better service for customers.
Additionally,Henry’s goal is to create a prescriptive framework that offers guidelines on effectively communicating explanations to users of AI systems. How users interact with a decision support system and process the information provided plays a crucial role in shaping their decision-making. This framework will be applied to help identify the best ways to convey the necessary information to operators.
-------------------------
Niels Scholten
“Working as a PhD researcher in the Responsible AI Lab at KPN has been both academically stimulating and very rewarding. Our lab focuses on a critical, high-stakes area within the AI community: responsible AI. My specific work centers on fairness—how to monitor and mitigate biases in machine learning models. This task, while essential, is a fine line to walk, especially when contending with the complexities of real-world data.
Early on in my PhD journey, I realized that only a small portion of academic research leaves a lasting mark on the field, and even fewer breakthroughs find their way into practical, decision-making models in industry. Driven by a desire to make a tangible impact, I strive to pose research questions potent enough to make meaningful contributions. At KPN, I feel that I have the opportunity to identify these impactful questions. In our lab, we don’t just theorize; we collaborate closely with KPN engineers and data experts to ensure our methods are applicable to real systems. This hands-on partnership is where I see my work making the most impact—transforming fairness from an abstract ideal into something that benefits users directly.
Of course, there's a noticeable gap between what academics research and what’s feasible in industry. This can be frustrating for both sides, as real-world limitations often clash with academic ambitions. But for me, this gap is where the excitement lies, as it’s precisely where my research operates. I’m constantly challenged to observe where theoretical approaches break down in practice and to find ways to bridge those gaps. This intersection of theory and application gives my work a tangible purpose that isn’t always easy to find in academia.
I feel fortunate to be in this position, especially since this wasn’t my experience during my master’s thesis work. As an academic, it can sometimes feel like once you publish your results, it vanishes in the void. Here, I can see firsthand how my research ideas intersect with real-world challenges. In the coming weeks, for example, we’ll be onboarding KPN’s various use-cases to our governance environment. For many data scientists involved, this will be their first opportunity to critically examine and report on the potential risks of their use cases, particularly from a fairness perspective. I’ll be assisting as an advisor on fairness, and I’m looking forward to the discussions and insights that will emerge.
Although this collaborative approach is immensely rewarding, it does come with potential challenges. Working closely with an industry partner means I can get caught up in into immediate, short-term “consulting” work rather than staying focused on long-term research goals. Thankfully, I have supportive supervisors—both academic and industry-based—who help me focus on my research. They remind me that my research not only serves KPN’s immediate needs but also provides a foundation for sustainable, long-term improvements.
As I continue this journey, my aim is not only to make rigorous, insightful contributions to the academic field but also to ensure that this work remains relevant in real-world applications. Ultimately, it’s about creating fairer AI systems that serve everyone—a goal I believe is well worth the effort.”